Client Alert
Containing Risk and Seizing Opportunity: The In-house Lawyer’s Guide to Artificial Intelligence
March 26, 2019
By
Robert P. Silvers,
Sarah Pearce,
Elena R. Baca,Thomas P. Brown,Scott M. Flicker,Emily R. Pidot,& Carson H. SullivanBy Robert Silvers (Co-Chair, PH Artificial Intelligence Group), Sarah Pearce (Co-Chair, PH Artificial Intelligence Group), Brad Newman, John Phillips, Elena Baca, Tom Brown, Scott Flicker, Emily Pidot, Carson Sullivan & Edward George
The rise of artificial intelligence (“AI”) and automated systems will be one of the defining developments of the 21st century. No industry will be untouched and AI is already driving tectonic shifts in sectors ranging from transportation to consumer technology to healthcare to financial services and insurance.
The legal impact of AI and automated systems is cross-border and cross-disciplinary. In-house counsel must be ready to support their companies’ product development, strategic acquisitions and partnerships, and data sharing arrangements within uncertain legal frameworks and rapidly changing technologies.
In this Paper, we outline critical considerations within some of the key legal verticals: data privacy and cybersecurity; intellectual property (“IP”) and trade secrets; fintech and financial services; employment law; trade controls; and AI governance, safety, ethics, and related litigation risks.
The legal landscape, and practitioners, will need to adjust to these new technologies—and quickly. Deals are happening now. Regulators are bringing enforcement now. Consumers, patients, and applicants for jobs and loans are being impacted by AI now. Facial recognition, autonomous vehicles, computer vision, augmented reality, and robotics aren’t coming soon; they’re already here.
As lawyers, we should lean into this innovation as we protect our clients against risks that are profound and even unprecedented.
I. What Is Artificial Intelligence?
The vast majority of existing AI advancements and applications are largely a category of algorithms known as machine learning: machines using and analyzing data to learn, reason, and act for themselves, making their own decisions, and improving upon them when faced with new situations, in the same way that humans and animals do. In other words—and at a very basic level—AI provides a means of giving computers behaviors similar to human intelligence.
II. Data Privacy and Cybersecurity
The data protection implications of AI are vast, particularly if we consider the multitude of legal regimes around the globe and those that are on the horizon. The California Consumer Privacy Act, for example, looks set to elevate privacy law expectations in the U.S. to a higher level and in a different direction, arguably towards those already in place in Europe under the GDPR. Depending on the industry, or the particular use of AI technology, there may be additional rules to consider. Financial services or healthcare businesses using AI applications, for example, will have to consider the regulatory frameworks specific to their industries, some of which are introducing AI-specific requirements. This expanse of disparate data protection laws around the world is one of the key challenges facing companies in the AI space today, and companies will have to ensure they comply with the laws in place in each of the markets in which they operate.
While the precise data protection issues will vary by jurisdiction and industry, we set out below a few of the key considerations, all of which are already attracting the interest of regulators and class action litigators.
Fairness, lawfulness, and transparency. The complexity of AI technology often means it is difficult for a company to explain clearly how it uses personal data—and indeed, the particular technique deployed may result in the use changing over time and becoming increasingly complex. In addition, it will often be difficult to identify a single lawful basis for the processing taking place, one of the key requirements of the European regime. How can a company rely on consent, for example, if it is unable to explain clearly the processing being performed now and in the future as the algorithm evolves? Such potential repurposing of personal data in unexpected ways, using complex algorithms that enable conclusions to be drawn about individuals with unexpected and possibly unwanted effects, could pose a threat to individuals’ personal data. The key here is to be transparent about what data is being collected and used— and how.
Data minimization. Most uses of AI rely on the collection and analysis of large volumes of data. Companies will inevitably be accused of taking their collection activities towards the excessive and may be asked to explain whether and why they are retaining the data for longer than may be perceived necessary—most likely to help improve and further replicate algorithms. Companies should anticipate these questions well in advance and consider how they will respond if called to account.
Rights of individuals: Businesses will have difficulty complying with the increased rights of individuals over their personal data after that data has been absorbed and processed within AI applications. How can the information be retrieved, extracted, and provided to the individual, for example? Again, transparency will be critical. Companies will also need to ensure they are developing their technology with such rights (particularly rights of access) in mind from the very start, building in appropriate security mechanisms. Put differently, the concept of “privacy by design and default” is real and needs to be implemented in a very practical way.
Cybersecurity also takes on profound importance in the AI arena, for at least three reasons. First, AI applications are often supported by vast pools of sensitive data. It will be critical for companies to assess the information security risks to their data and to implement reasonable and effective controls, accounting if necessary for industry-specific regulatory requirements as appropriate (within financial services or healthcare regulatory frameworks, for example). Second, manipulations of AI training data can impact the quality of algorithms and the decisions they produce. This is known as “AI poisoning,” and, in addition to creating litigation and regulatory risk, it can severely undermine customer confidence in a company’s products and services. Companies will therefore need to ensure they are protecting the integrity of their training and algorithmic development processes with the appropriate security controls and procedures. Third, companies need to account for the security of autonomous systems to ensure their safe operation in the physical world. Poorly secured autonomous vehicles or drones, for example, can create significant risk to human safety and property and attendant liability. Companies will need to ensure these autonomous systems are protected from malicious attacks that can compromise their safe operation.
Data protection issues are real and serious, but provided they are properly managed, the applications of AI can thrive without jeopardizing individuals’ rights over their personal information. It may be possible, for example, to use techniques that alter the state of data, rendering personal data truly anonymous through established de-identification processes. Such measures can substantially mitigate core data protection concerns.
Ultimately, companies should ensure they implement a robust and accountable privacy and security governance framework that is tailored to their particular environment, AI application and regulatory regime. Such a framework will include appropriate notices and policies and procedures around the collection, security, and use of data—particularly personal data—in their businesses.
III.Technology Transactions and Intellectual Property
The IP implications of AI cannot be overstated. IP-intensive industries support at least 45 million U.S. jobs and contribute more than 38.2 percent of U.S. gross domestic product. The increasing prevalence of AI presents novel and complex questions regarding ownership and infringement of AI generated IP. It also requires new ways of approaching technology transactions and drafting and negotiating IP contracts and renewed focus on accounting for and protecting trade secrets.
For example, the majority of AI technology is currently implemented as software running on off the shelf computer hardware. Contracts to develop such software must account for ownership and licensing of pre-existing IP incorporated into the AI system, and need to include properly drafted indemnities related to infringement of third-party IP rights. Licensing and transaction agreements must also cover infringement by the AI applications themselves. Since AI is not legally a person, under the current law it cannot be liable for IP infringement. Therefore, the person or entity that controls the AI will likely be held liable for any infringement.
Additionally, in cases where a seller or manufacturer creates an AI system that makes use of the buyer’s data, questions arise as to who owns the rights to the underlying data, and who will own the new data associated with ongoing application of the AI system. These highlighted concerns are by no means exhaustive, and IP contracts for AI applications will require new thinking and innovative drafting.
The question of who owns the IP becomes particularly tricky in two main circumstances: (1) where one party creates the AI for a second party to use for purposes of generating new IP, or (2) where the AI application created the IP independent of human action and/or exceeded its intended programming. There are questions as to whether traditional IP legislative frameworks, be they in the U.S., the U.K. or elsewhere, can properly account for IP created solely by AI. And while the U.S. Congress, for example, is considering proposed legislation that would dramatically alter the traditional IP landscape by creating a separate framework for AI applications and IP, it is imperative that companies avail themselves of the most creative and deep understanding of the current legislative framework.
It is not only in the context of technology transactions that companies must position themselves to take unprecedented steps to minimize the risks to IP presented by the increasing adoption of AI applications. New modes of proactive and strategic thinking, which we are helping our clients develop, are also required when prosecuting and defending against allegations of infringement by AI applications.
Finally, identifying and adequately protecting company trade secrets in this shifting AI landscape merits increased attention. The algorithms that comprise the basic components of AI applications are not the only confidential and competitively valuable information requiring affirmative protection measures. Neural networks and associated AI learning require mass amounts of confidential data in order to function properly. What that data consists of, how it is generated, the ways in which the AI applications process and learn from it, and the new information created by the relevant AI, all involve embedded trade secrets that must be recognized and safeguarded. For well over the last decade, we have a track record of working with the world’s leading AI producers to assist them with this mission-critical task.
IV. Financial Services and Fintech
The financial services industry has been grappling with legal issues arising from automated decision making since Bill Fair and Earl Isaac teamed up to found Fair Isaac & Company, now known as FICO, in San Rafael, CA in 1956. The consumer credit and insurance industries served as proving grounds for their shared insight that data properly structured and analyzed can help businesses make better decisions. Concerns about the “fairness” of decisions made by algorithms in those industries prompted the passage of legislation (in the United States and elsewhere) to address those concerns more than 40 years ago. The core challenge with algorithmic decision making in the financial services industry is reconciling the predictive power of information with concerns about privacy on the one hand and fairness on the other.
Information about consumers is predictive. For companies trying to predict whether a given consumer will repay a new loan, predictive information can be both negative and positive. If a consumer has declared bankruptcy in the past, that consumer is less likely to repay a loan than someone who has not. On the other hand, a consumer who has a history of staying current on outstanding loans over an extended period is more likely to repay a new loan than someone who does not have such a history. The fact that information is predictive gives rise to a complex set of incentives for consumers and for companies serving them. Consumers with negative signals in their history would prefer that their information not be collected or revealed to those organizations making decisions about them. Conversely, consumers with positive histories have an incentive to find ways to share their information, even quite sensitive information, with such organizations.
The predictive power of information (including the algorithms that synthesize and analyze the information) cuts against widely shared concepts of fairness. The willingness and ability to repay credit is not distributed evenly across populations of consumers. And underwriting algorithms do not judge each prospective subject on his or her own merit. That is, algorithms explicitly use information derived from the performance of others to decide whether a given subject is likely to do whatever the algorithm is intended to predict. These decisions are fraught given that they take place against the backdrop of a legal regime that long discriminated against large segments of the population in employment, education, and the general distribution of government benefits based on race, sex, and other demographic characteristics.
These issues came to the fore in the late 1960s and early 1970s as the first information technology boom made it possible for banks to automate the process that they used to underwrite credit. These widespread concerns prompted an evaluation of the regulatory framework for consumer financial services through a bipartisan effort that began under President Johnson and continued under President Nixon. That effort culminated in the adoption of a host of laws, including two that continue to govern how information is collected about consumers for use in underwriting and how algorithms make decisions about consumers: the Fair Credit Reporting Act (“FCRA”) and the Equal Credit Opportunity Act (“ECOA”).
The primary challenge for companies today is reconciling existing regulatory obligations with tools that are much more sophisticated than the simple linear regression models developed by Fair and Isaac. For example, both the FCRA and ECOA require financial institutions to explain the basis of adverse decisions to consumers. This was easy when underwriting models used a small number of variables to make predictions and when the models assumed that the variables behaved independently of one another. With models now using thousands of variables and models being built that anticipate co variance among those variables, communicating the precise reason for a decision to deny credit (or to price credit in a particular way) has become quite complex. Similar challenges arise in defending new modeling techniques against claims that those techniques simply aggravate disparities in the distribution of credit.
We have unparalleled experience in helping firms manage these issues—often even persuading regulators that new credit underwriting technologies can be deployed in a way that mitigates, rather than aggravates, concerns about equitable credit distribution.
V. Employment Law
Artificial intelligence has already begun to transform the workplace and add complexity to traditional employment concerns. Its use heralds tremendous change in the areas of talent acquisition and development and will significantly impact recruitment and hiring, promotions, firing, and internal and external oversight of employees. A recent McKinsey study determined that up to 800 million workers worldwide may lose their jobs to AI by 2030, and half of contemporary work functions could be automated by 2055. AI is already being used to staff fast food drive-through windows, determine the ripeness of produce before picking it, design clothing, and take calls at customer service centers. Assuming the workplace disruption and displacement caused by AI applications occurs as predicted, it is almost guaranteed that AI employment legislation will be enacted. Until that occurs, employers should be cognizant of the shifting landscape and the rapidly evolving new challenges related to AI’s potential impact on the employment landscape.
Recently, employers have begun to use (mostly third-party) AI applications for recruitment and hiring. Many new-to-market vendors profess the ability to help employers source the best prospective candidates and job applicants and to predict job success based on their algorithms and technology. They offer a range of AI-related services, including screening resumes, conducting on-line soft-skills assessments or personality tests, and analyzing video interviews. Currently, AI’s primary intended benefit is increased efficiency and, it was thought, to eliminate bias in the workplace. While efficiency will likely be improved, the employment-law results may prove surprising.
AI applications in this space have been, and continue to be, marketed as a means to reduce the risk bias, whether unconscious or conscious, might result in decisions that conflict with an organization’s goal of selecting and rewarding solely based on merit, skill, and talent. The marketing of such products stresses AI’s “ability” to assess candidates and employees with a high probability of success while eliminating human bias. However, such blanket assumptions have recently proved problematic. Notably, many vendors selling AI-based recruitment and hiring solutions claim to have “validated” their tools, but even a cursory review demonstrates that the “validation” would never conform with accepted legal guidelines (the Uniform Guidelines on Employee Selection Procedures), nor protect the employer from adverse impact hiring claims (likely in the form of a class action lawsuit). It is crucially important that employers and their legal counsel vet these companies and their promises carefully, including a review of the contracts for services (which most likely do not contain indemnification provisions) before retaining these vendors. At least in some cases, AI has already reflected its own inherent biases. For example, in October 2018, Amazon scrapped the use of its AI recruiting technology after discovering the technology was not acting in a gender-neutral way. Thus, at this stage, companies need to retain critical human oversight of the recruiting function, generally, and carefully scrutinize the efficiency and potential bias of AI applications.
In today’s globalized competitive environment, retaining top-level talent is ever more critical. Producers of existing AI applications seek to justify the acquisition expense of their products based on the assumption that machines are better than human managers are at identifying and assessing high and low performers, which, in turn, furthers both retention of good employees and performance tracking and management of underachieving workers. According to a 2017 study conducted by The Center for Generational Kinetics, “just 47% of managers use any type of data at all when making salary or promotion decisions.” There is no question that AI applications empower companies to use “hard” data sources, like salary increases, skill sets, and performance ratings, to predict future high performers, encourage employees who are struggling, and recognize negative situations. AI applications can also assist the HR function in analyzing and acting upon job-specific performance data by identifying the behavioral preferences that are most conducive to successes in a particular position.
AI is also being utilized in the context of performance management analytics by monitoring and collecting data on employees. Companies will need to ensure they implement such technology in a manner that is compliant with data privacy and workplace legal frameworks in the jurisdictions in which they operate. Once adopted consistent with these parameters, such data can be used to determine engagement, productivity, and absenteeism and how these factors impact performance. In the near future, it may be routine for companies to utilize AI applications in this manner to decide whether to retain, promote, or terminate an employee.
However, there is risk. AI-based solutions meant to curtail labor costs have been met with some degree of public scrutiny. A good example is the public response to retail employers’ use of computerized staffing algorithms to determine employees’ work schedules. The algorithms determined labor needs based on the weather, the flow of customers, and sales. Employees would be placed on “on-call time” or “just-in-time” schedules, and the algorithm would determine who was called into work just hours before the potential shift. Workers’ rights groups decried the unpredictability of on-call scheduling practices, given that employees under these schedules were required to be available for shifts (and thus, refuse other work opportunities, arrange for childcare, etc.), but then risk not actually being allowed to work nor be paid for those shifts. With the public scrutiny of these policies, interest from state attorneys general, and hearings in local and state legislatures throughout the country, many prominent retailers have ended the use of on-call scheduling. As recently as January of 2019, the New York State Department of Labor issued proposed statewide regulations that would require employers to pay employees during “on call” shifts, even where the employee was not asked to work during the shift, thus limiting the potential benefit to employers’ labor costs by using the predictive AI.
The increased prevalence of AI applications being utilized in the traditional Human Resource function effectively guarantees that employment-based disputes will arise and result in litigation—as well as create internal employee concerns and potentially adverse external publicity. When, and in what manner, humans should remain in the chain and the recommended oversight required for the use of AI applications in the work context can present vexing questions.
VI.Trade Controls
AI-related technology is growing more important in areas that are sensitive from a national security standpoint, including military applications, cybersecurity, drones and robotics, and critical infrastructure. AI capabilities developed for benign, consumer-facing uses may also have more sensitive applications. And the escalating “tech arms race” between the U.S. and China has national security dimensions beyond the traditional economic and trade competition between the two countries. We are already seeing national governments react by extending their traditional trade controls authorities to regulate how AI can be acquired by, or exported to, foreign companies—even in what appear to be purely commercial contexts.
Most prominent among these movements is the U.S. Export Control Reform Act (“ECRA”), enacted last fall as part of the defense authorization package for 2019. Under this law, an interagency working group, including the Department of Defense, has been empaneled to identify and establish heightened controls on the export, re-export, and transfer of so-called “emerging and foundational technologies.” Regulators have preliminarily identified broad categories of AI and machine learning technologies as candidates for these heightened controls, including: neural networks and deep learning computer vision (e.g., object recognition, image understanding); decision support and teaching systems; and speech and audio processing AI cloud technologies and AI chipsets. Once so classified, significant elements of hardware, componentry, software/code, and documentation embodying or disclosing these technologies could become subject to stringent export licensing and other restrictions, including on transfers to China or Chinese nationals.
These restrictions, if implemented, could have a transformational impact on U.S.-based R&D centers, international technology joint ventures, and cross-border supply chains serving the developing AI industry, as business models dependent upon tight integration of U.S. and non-U.S. research activities could become impractical virtually overnight.
As regulators tighten controls on what technology can leave their countries, dealmakers across the globe are also feeling the tighter scrutiny of foreign investment review boards, which assess proposed inbound investment for national security risk. Governmental panels like the Committee of Foreign Investment in the United States (“CFIUS”) and Australia’s Foreign Investment Review Board have sharply escalated their investigations into proposed investments.
We expect these reviews to focus closely on deals involving acquisition of AI-related technologies, particularly where the acquiring party is Chinese or has extensive ties to China. We also expect CFIUS and similar bodies to continue viewing large pools of personally identifiable information as sensitive from a national security perspective. CFIUS, for example, has already blocked or imposed significant conditions on a number of deals involving large personal data pools. With data being the key driving force behind AI development, a number of potentially attractive investment targets may find themselves subject to national security reviews, even if those targets themselves are not involved in AI-related technology or innovation. Early in the due diligence phase, dealmakers will need to account for these broader and more intensive government review processes, both to assess deal risk and to ensure development of realistic execution timetables.
VII.AI Governance, Safety, Ethics, and Related Litigation Risks
The impacts of AI are reaching deeper into consumers’ lives and spreading to more corners of industry. Companies will be called on to explain the fairness and consistency of the decisions their algorithms render. They will face scrutiny over whether their AI-driven services are delivering outcomes free of bias on the basis of gender, race, and other protected categories. They will face fundamental quality-control questions: are the outcomes “right” and, in certain contexts such as autonomous vehicles, are they causing injury to people and property?
The tension between machine-driven decision-making and the legal system’s insistence on accountability is real and will only grow. Litigants and regulators alike will be pursuing these nascent but profound questions in earnest. It is inevitable that the new competitive business landscape fueled by companies that incorporate AI as a driver of revenue will likely trigger a new frontier in the area of business disputes and class action lawsuits. For example, AI will accelerate product design and functionality across many industries, including medical diagnostics and therapies, smart phones, consumer goods considered part of the larger family of “internet of things,” material design, retail, and telecommunications, particularly as carriers move to the 5G platform and build out new offerings and services.
As AI becomes a more integral part of the decision-making process for how to develop these products and services, future lawsuits will focus on (apart from traditional product liability concerns) whether the products’ ultimate performance meets consumer expectations created by robust marketing and advertising strategies, many of which will likely become automated in order to address the rapidity of change in the marketplace. Those same strategies will come under added scrutiny as AI is used to identify market gaps, which will be exploited through the development of more refined marketing and advertising material developed for online platforms. Those strategies often lead to detailed critiques in various blog postings that form the basis of class action lawsuits, in addition to triggering potential claims based on violation of existing or new consumer protection laws.
AI also will be used by sellers of consumer products to develop lending strategies and other potential financial products that are created to boost sales and to capture market share. These new types of financial services will not only face regulatory scrutiny, but will also surely be exploited to form the basis for class actions or other business torts and similar legal action.
As noted above, data development and storage will continue to surge, triggering divergent and conflicting uses. These issues raise many traditional privacy concerns and will spawn new rounds of litigation. States will enact separate statutory schemes, which will trigger unique liability issues and place increased pressure on in-house counsel to manage related litigation risks and business strategies, as further discussed below.
Apart from consumers, companies will collaborate on AI implementation across international platforms, leading to a whole host of licensing agreements and business contracts that will require a new set of skills to pursue effectively AI-based claims against a competitor that fall outside the traditional patent or trade secret dispute. Disputes that arise between companies in different countries will lead to increased use of arbitration and create opportunities for creative dispute resolution strategies and a greater need for in-house counsel to manage the related business risks.
To protect against civil liability, regulatory scrutiny, and reputational harm from unintended outcomes across a wide-variety of AI usage, companies need to support their AI strategies with governance structures designed and overseen by humans. The components of an effective governance strategy will differ by industry and application, but key pillars can include:
Robust quality control protocols to test that those algorithmic decisions are producing outcomes that can be fairly expected by companies and their customers. The deployment of AI throughout numerous industries will put strain traditional infrastructure and systems that exist to manage litigation risks across key business platforms and create an urgent need for new protocols. These protocols should be risk based; more consequential applications (e.g., decisions impacting healthcare or personal finance) should be more tightly overseen than those with less impact on consumers (e.g., recommendations for products or entertainment content that a consumer might like based on prior purchases).
Policies and procedures to reduce the risk that algorithmic training data at the front end and outcomes at the back end are infected by unintended biases, particularly in the consumer, financial, housing, and employment contexts.
Commitments to transparency and accountability. Companies should consider when and how to give notice (to consumers and business partners) that decisions impacting them will be made on an automated basis. Companies should also consider creating procedures for redress when a consumer believes he or she has been treated unfairly by an algorithmic decision-making process.
Internal qualifications requirements for AI operators. This will demonstrate responsible oversight by ensuring that only qualified individuals with the right technical competencies are employed in AI-related positions.
Statements of values and ethics. Just as companies often express the general principles that drive their businesses, an increasing number are now articulating those principles that animate and surround their development and deployment of AI. This can include statements emphasizing commitments to quality outcomes, consumer privacy, safety, unbiased and fair decisions, and transparency around the use of AI.
VIII. Conclusion and a Look Ahead
The economic and societal benefits of AI will be profound, but so too are the risks and people’s concerns about how automated processes will impact them and their families. As companies deploy AI-driven services and products, they need to expect that these risks and concerns will manifest in various forms of litigation, regulatory enforcement, and protest, placing a premium on the ability to understand and explain artificial intelligence and the decisions that the owners of a business make to implement this technology.
Creating and constantly refining sound governance principles at the ground level will help companies answer these concerns and comply with existing legal regimes—and ultimately mitigate their potential for undermining business objectives and triggering legal liability. Conducting this front-end compliance and governance work under the attorney-client privilege is an essential process to ensure that businesses can ask the hard questions, make the difficult risk-based decisions, and proceed with confidence.
Please contact any of the authors, or your usual contact at Paul Hastings, to ask further about the ways we are helping our clients navigate their AI-related development, growth, and compliance strategies.
Contributors
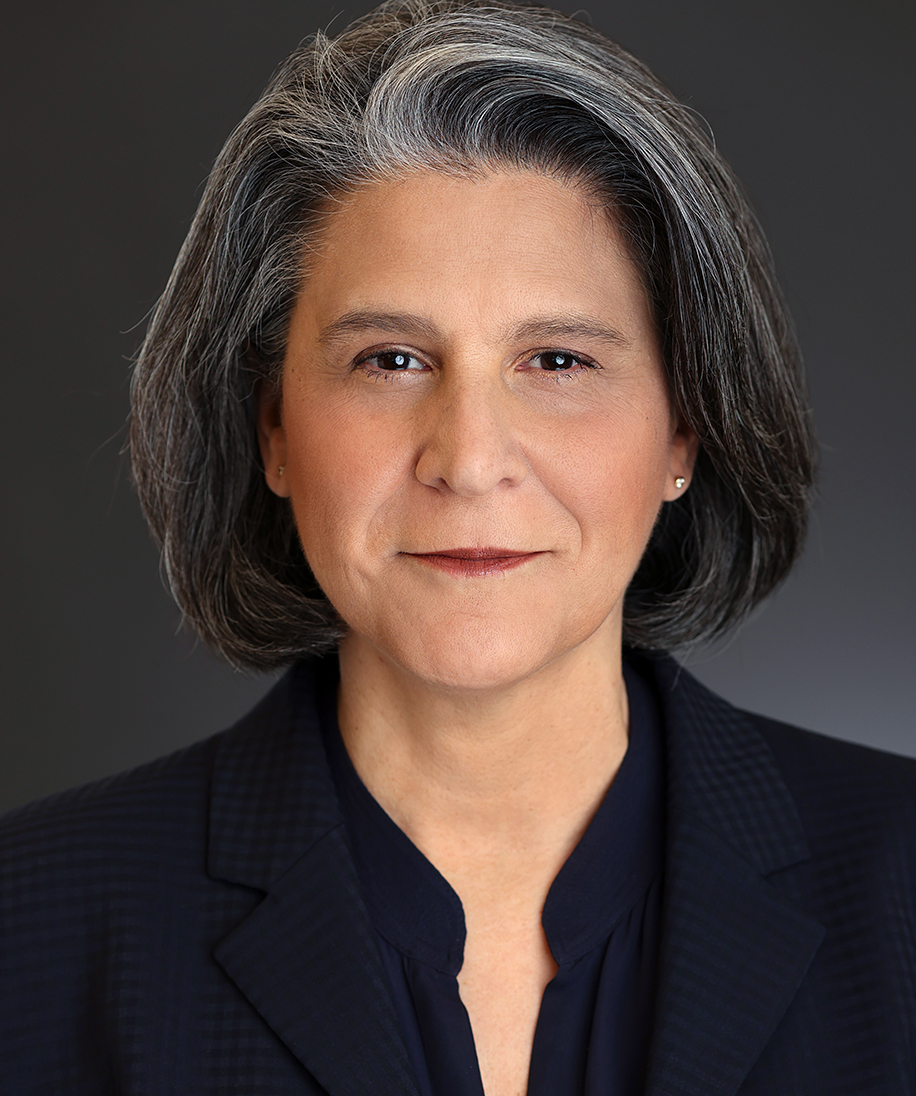
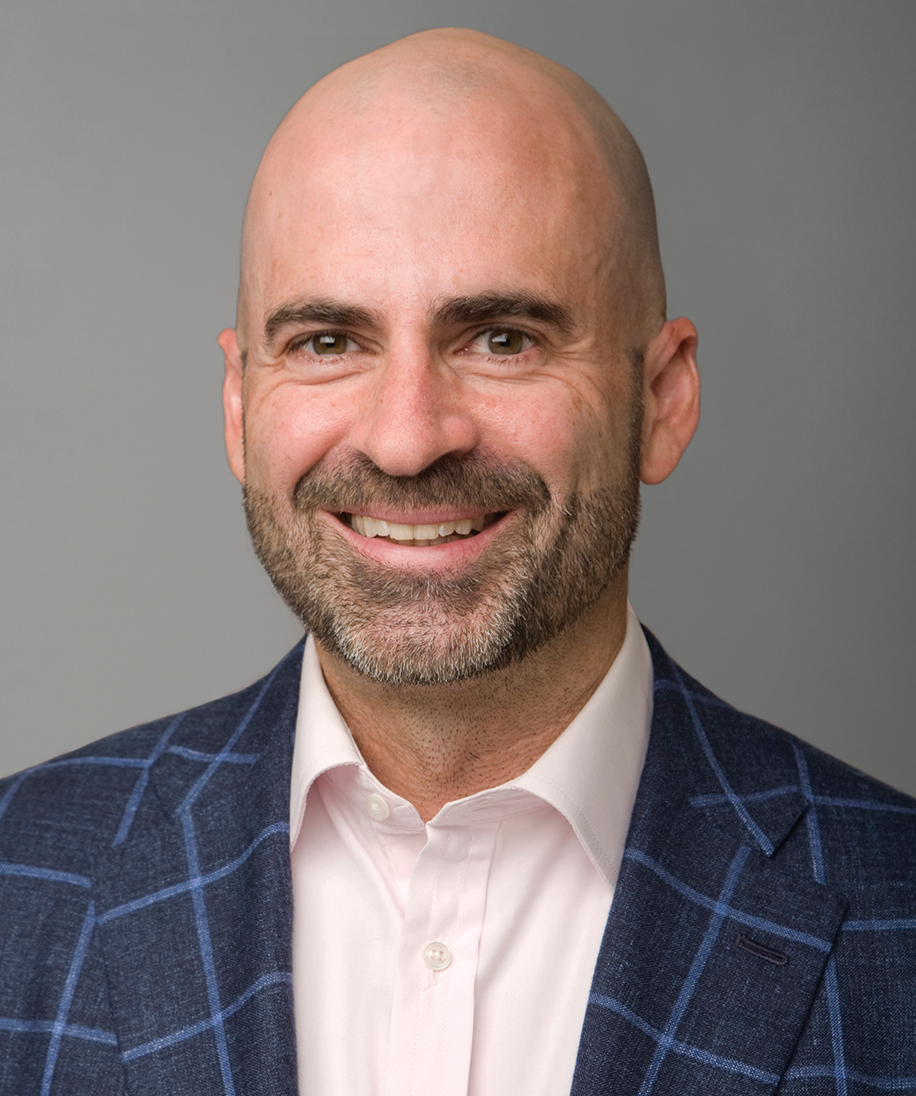
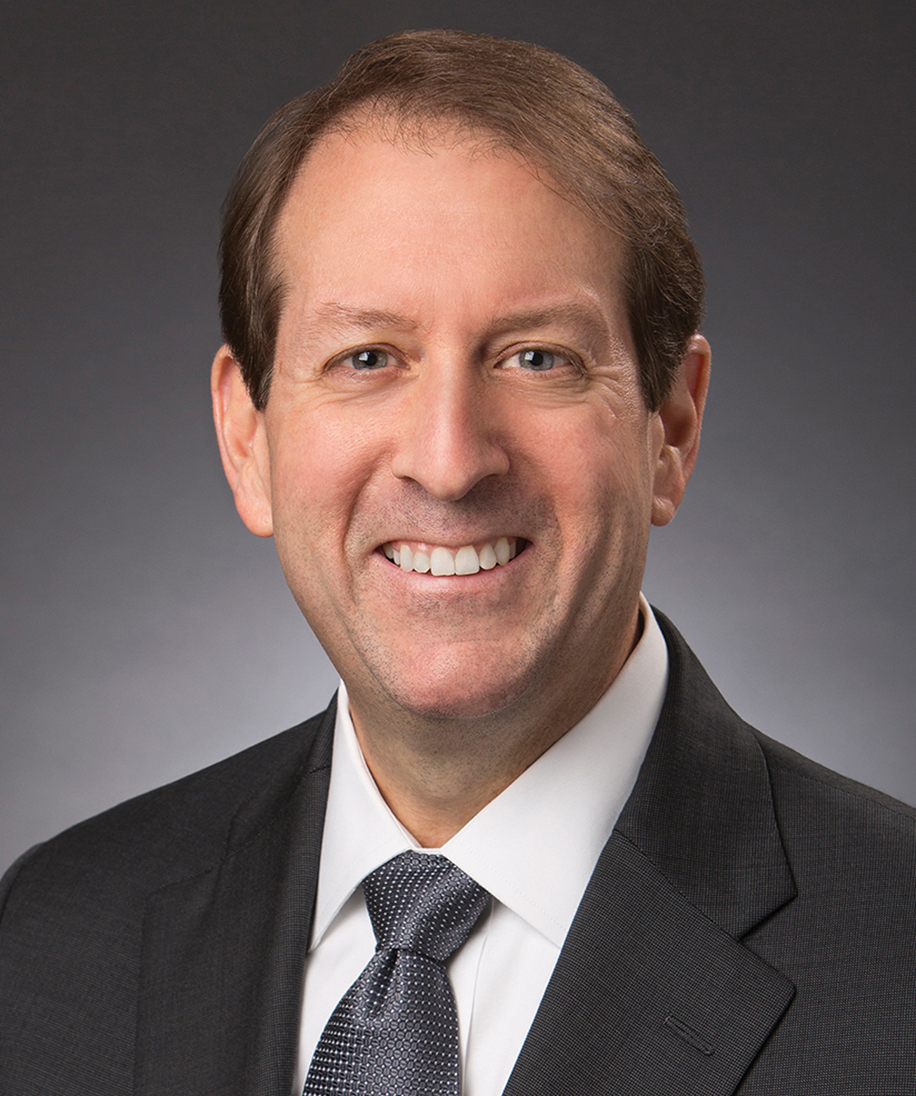
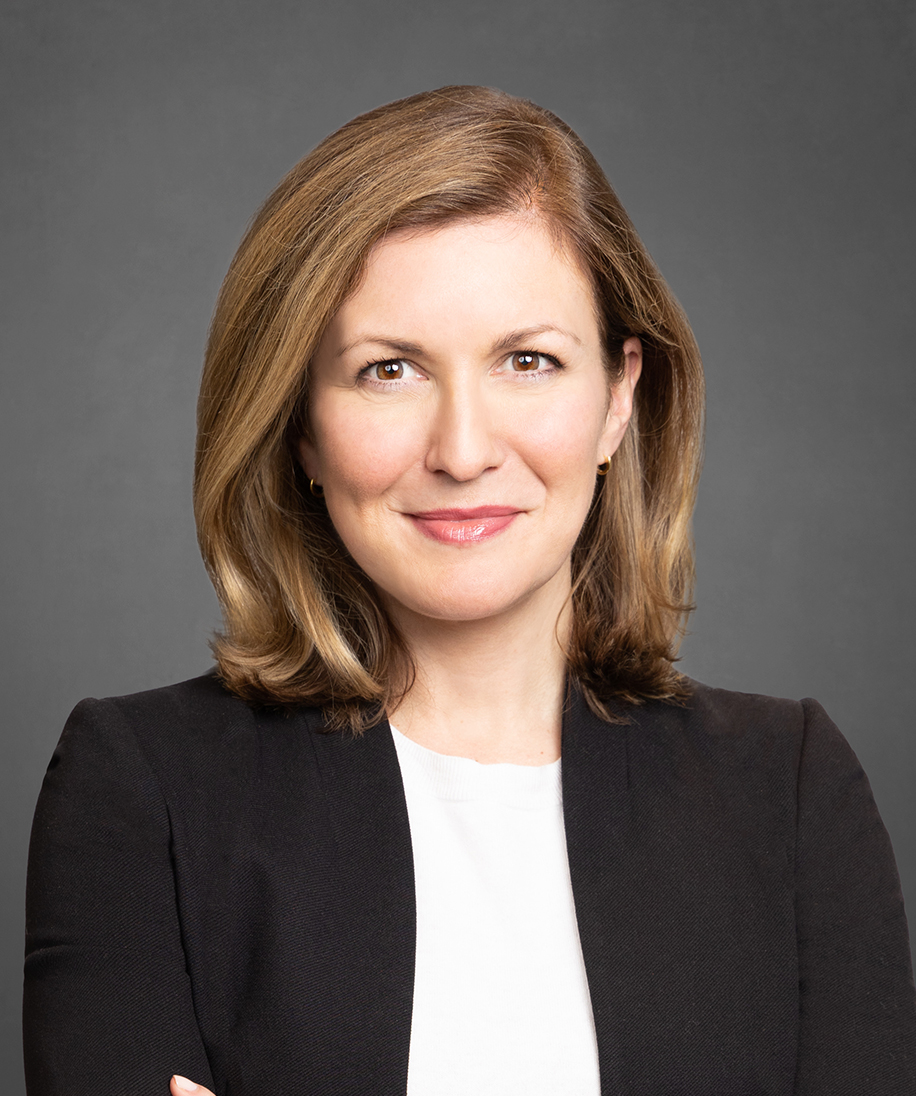
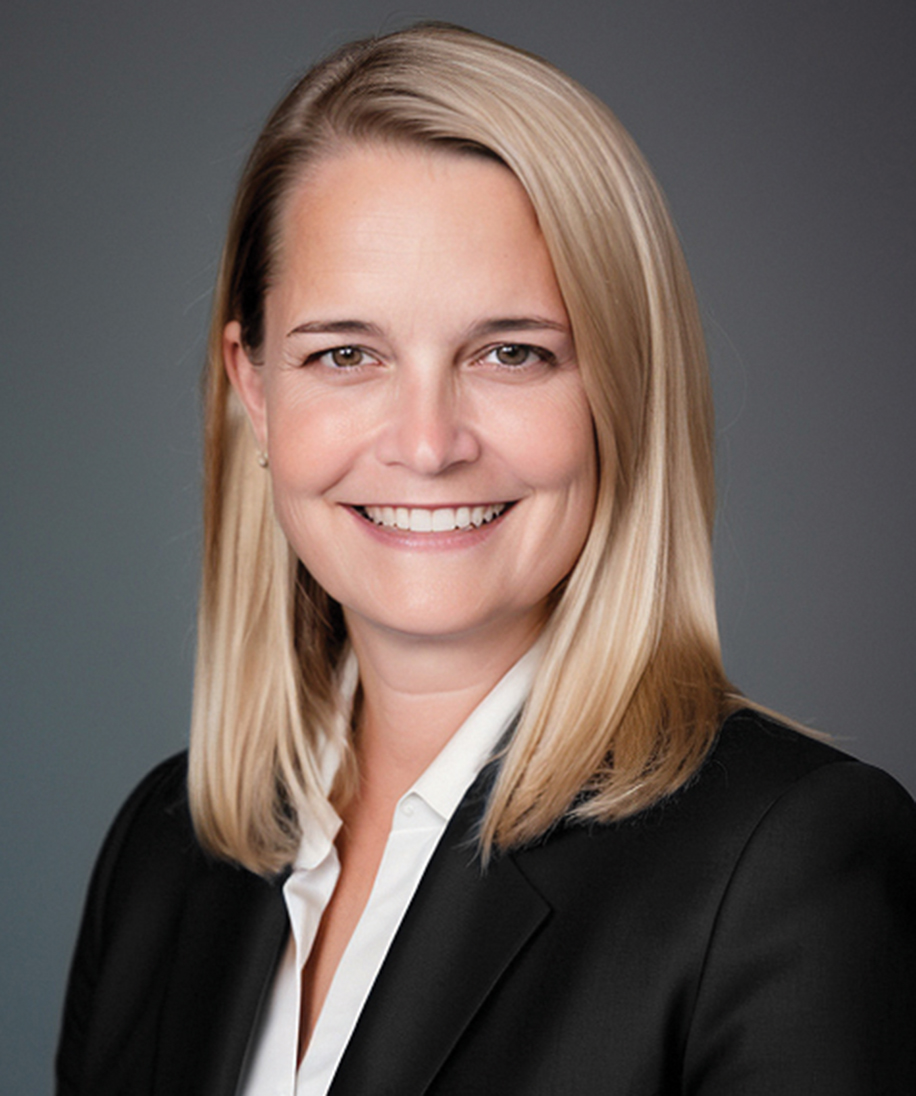
Practice Areas
For More Information
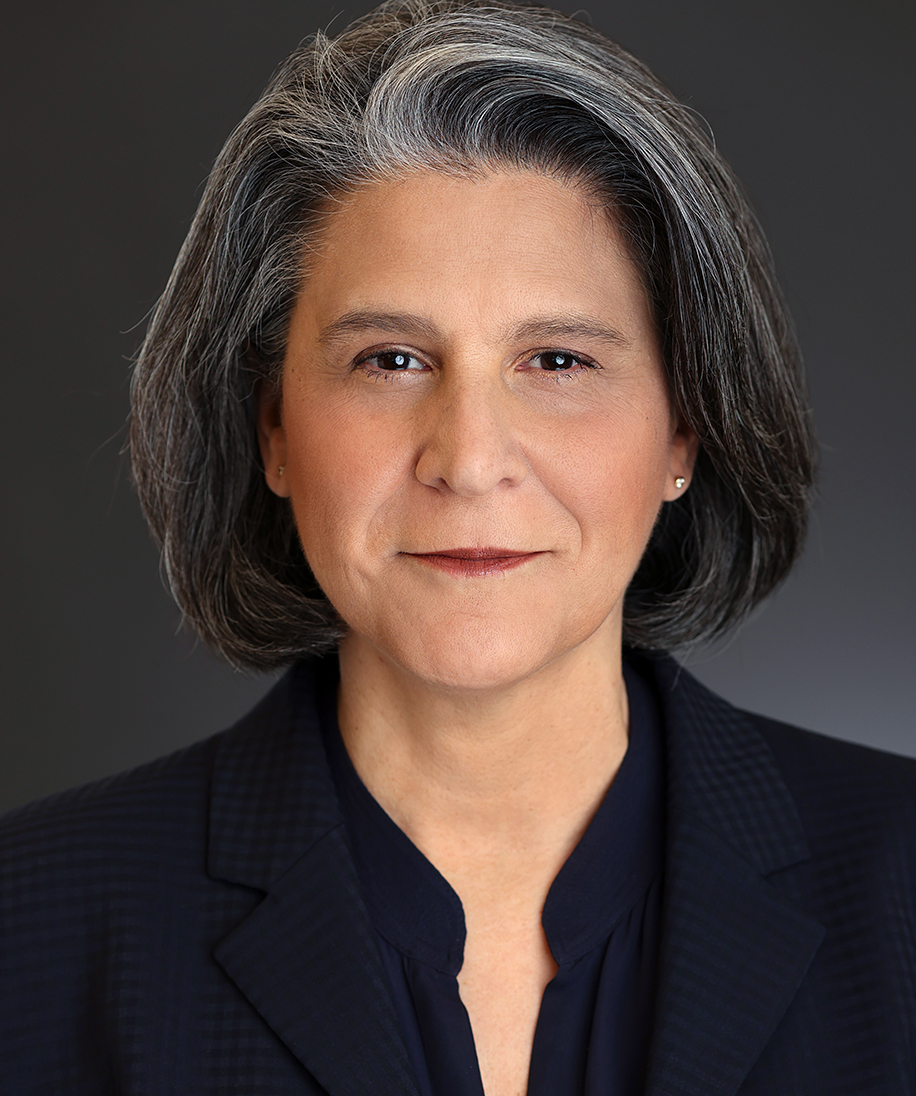
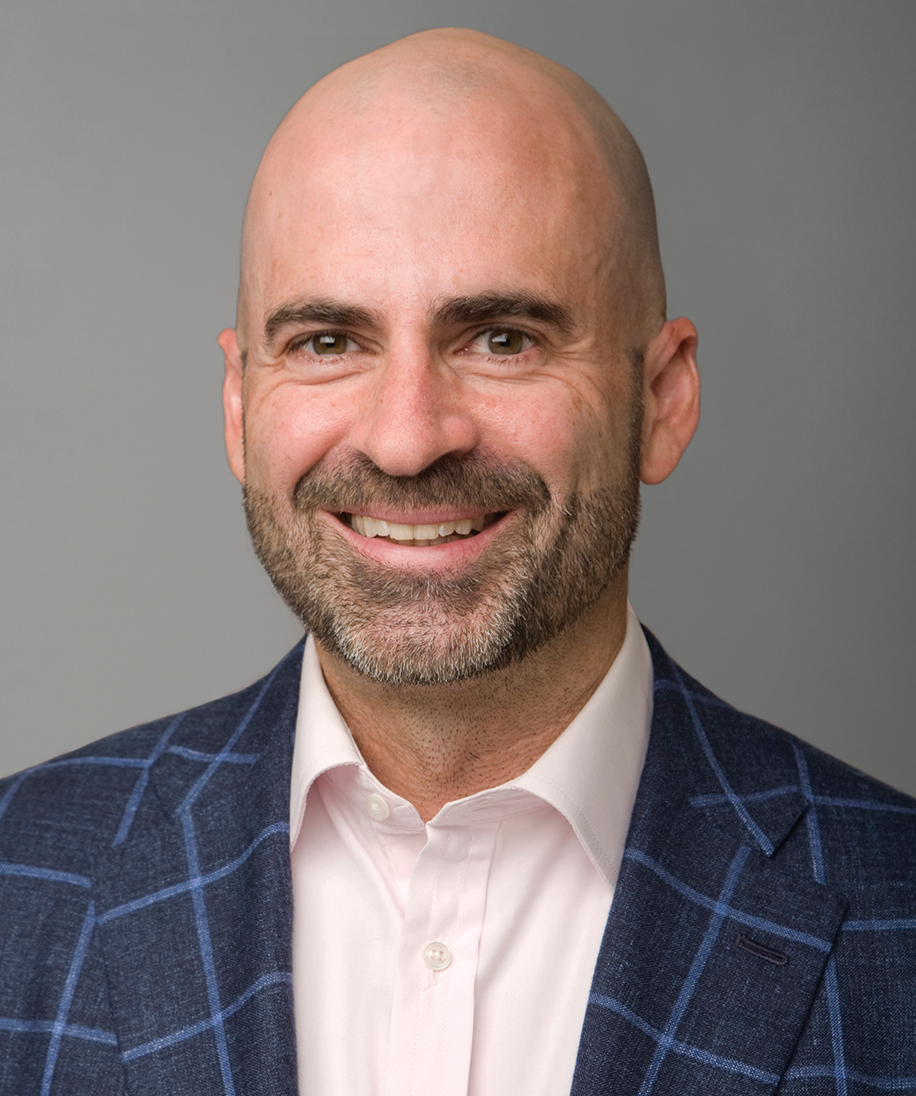
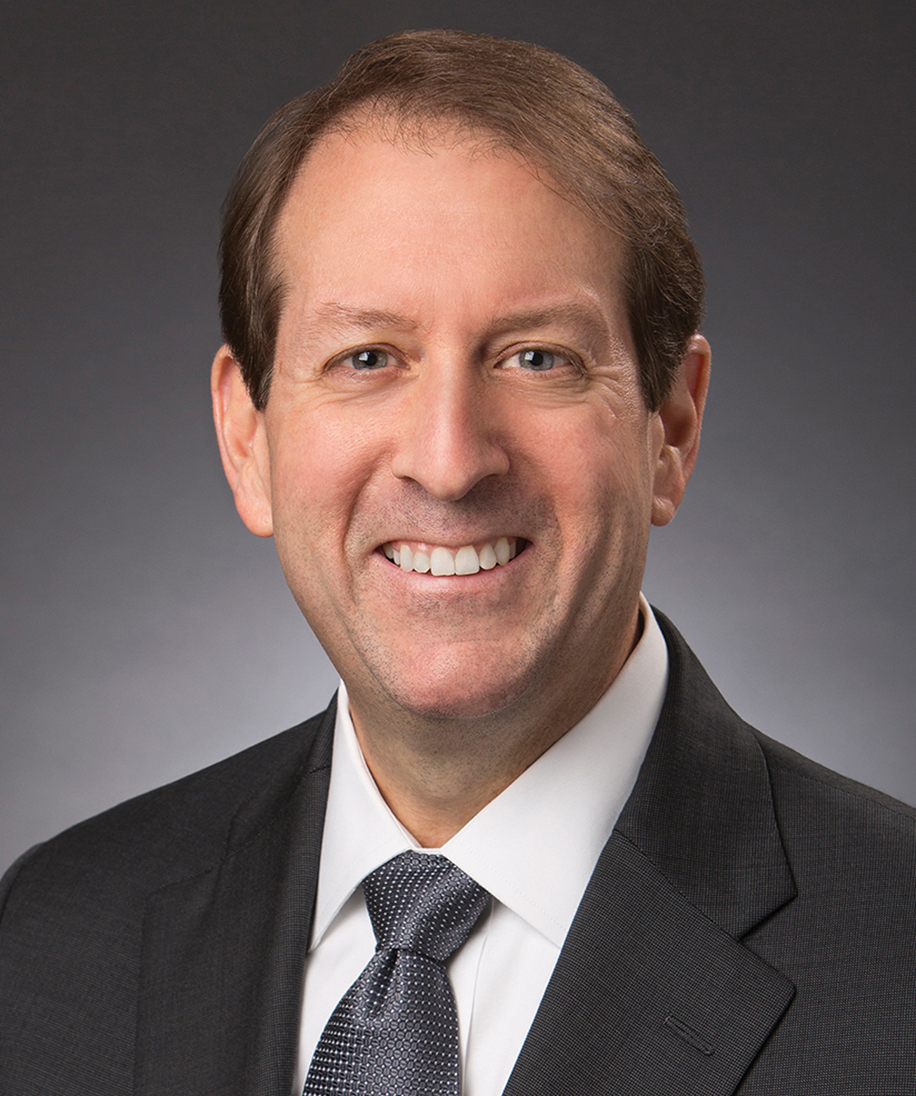
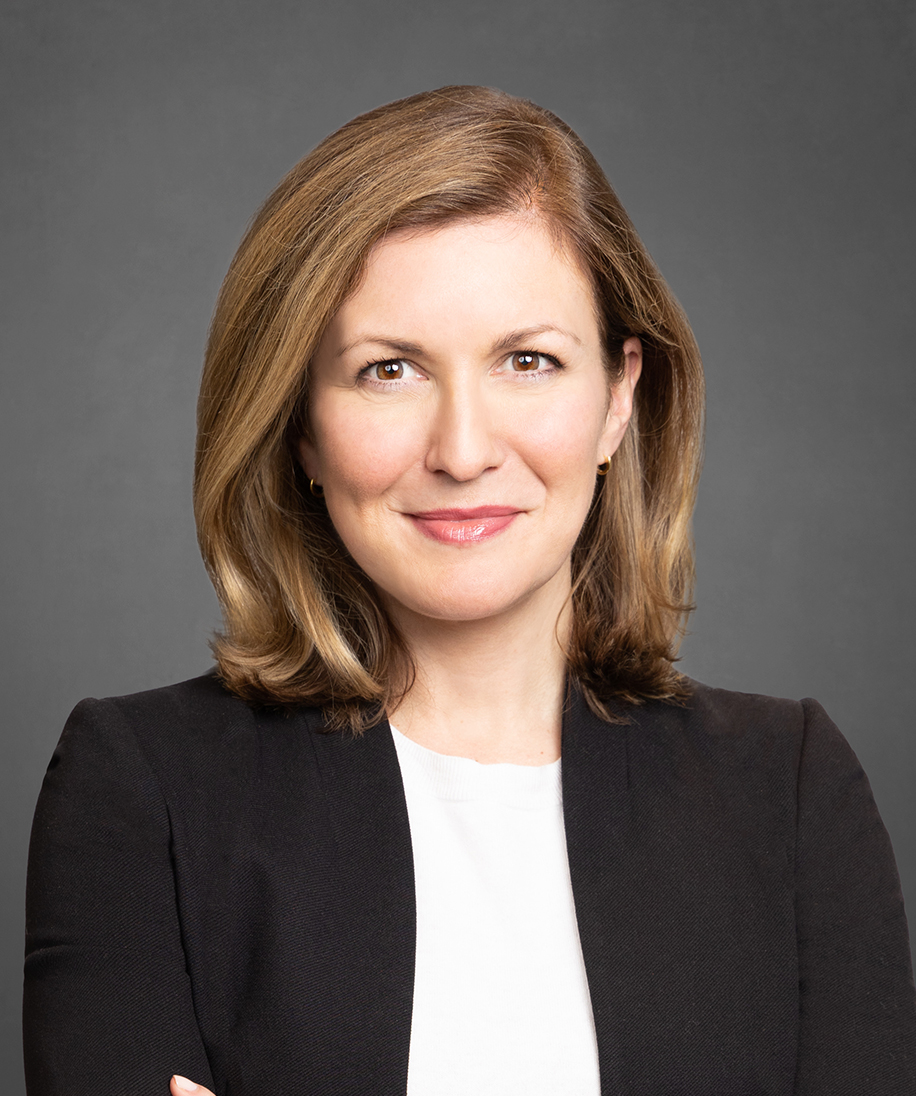
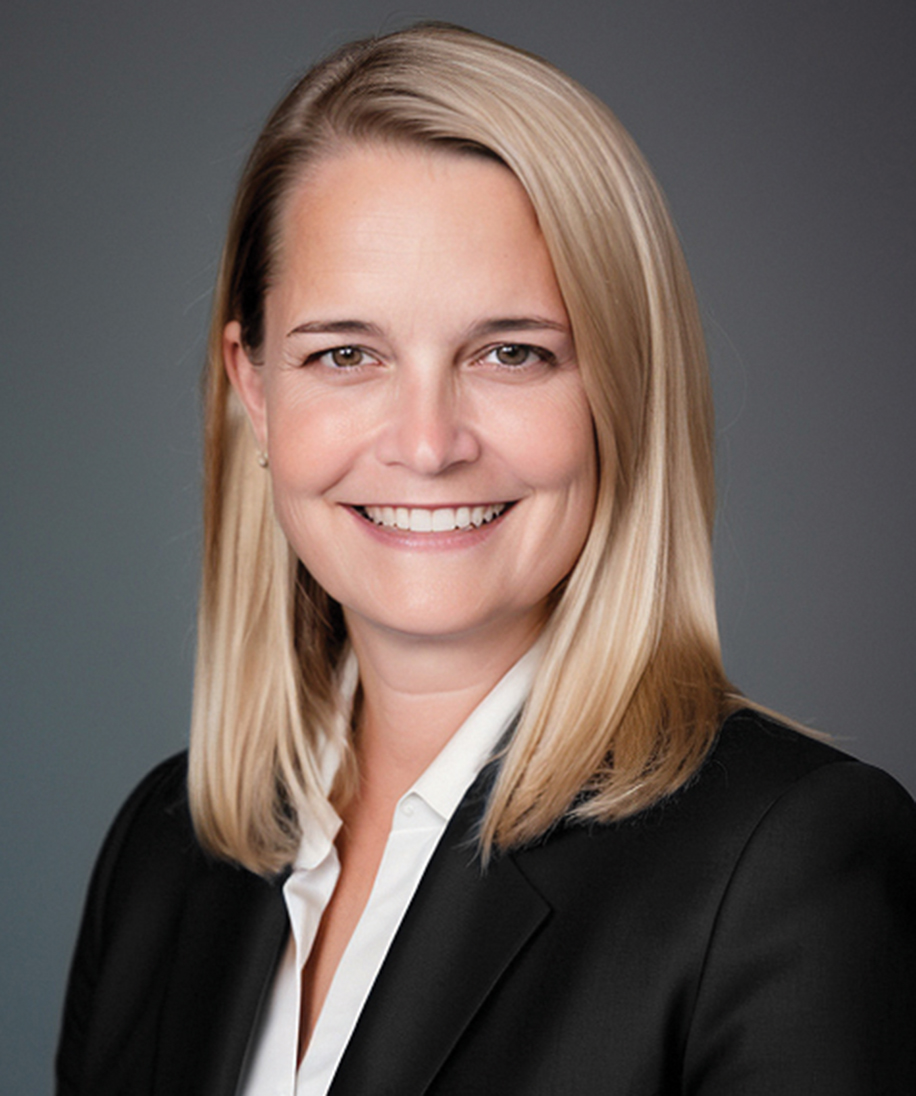