Client Alerts
Intellectual Property Considerations for AI Companies: A Guide for Investors and Startups
May 29, 2024
By David Ambler,Aaron Charfoos,Lisa Nguyen,Todd M. Schneiderand Jason Raeburn
Introduction
In the rapidly evolving landscape of AI, the valuation and viability of AI companies are extensively tied to their intellectual property assets. For AI companies, safeguarding these assets is not just about legal protection—it’s about securing a competitive edge and enhancing market value. For investors, understanding the IP landscape is crucial to assessing both the risks and the growth potential of their investments. This discussion delves into the complexities of IP evaluation, offering both AI enterprises and their investors a roadmap to navigate the intricate legal frameworks, identify potential risks, and capitalize on the strategic growth of AI enterprises.
How AI Companies Create AI
Most AI companies harness vast datasets to train algorithms, enabling their systems to perform tasks ranging from simple classification to generating complex content. This training process can involve collecting, processing or analyzing data from various public and proprietary sources, often leading to complex legal and ethical considerations.
Understanding the Legal Framework
The legal landscape surrounding AI and IP is nuanced and continually evolving. Key areas of concern include the legality of data acquisition methods, such as scraping public websites, and the use of such data in training AI models. The legality of these practices often hinges on the specifics of how data is gathered and used, raising potential compliance issues with copyright laws, terms of service of the data sources, as well as various statues relating to the access and use of computer systems (which can vary based on jurisdiction).
Infringement Risks in Training AI
Utilizing copyrighted materials for training AI poses significant legal risks and potential litigation. The core legal challenge for AI companies involves determining whether the use of copyrighted training data or the outputs of AI constitutes infringement of the underlying copyrighted works and, if so, whether such infringement may be permitted as “fair use” (or, depending on the jurisdiction, whether any defenses or permissions apply to the activity at all). The legal landscape here remains unsettled, with ongoing cases across the globe potentially setting important precedents and defining market practice.
Comprehensive Examination of IP Assets
A meticulous review of IP assets and an awareness of risk is essential. Below are key areas of focus:
- Ownership and Assignment
- Employee and Contractor Contributions: AI companies often deal with unique and advanced algorithms that can blur traditional IP boundaries. AI companies must secure intellectual property developed by employees and contractors. It is vital to ensure employment contracts and independent contractor agreements robustly address IP ownership rights, including explicit IP assignment clauses that properly contemplate the nature and scope of the activity being conducted. These agreements should cover not just software code, but also data sets and machine learning models, ensuring that all facets of AI innovation are owned by the company.
- Prior Agreements and Encumbrances: Reviewing previous agreements is crucial for identifying any existing IP encumbrances that might impede the company’s operations or affect IP ownership. This includes checking for any residual claims by previous partners or collaborators that could affect the company’s full ownership of its AI developments.
- Licensing Arrangements
- Inbound Licensing: Thoroughly analyze all licensing agreements where the AI company uses third-party technologies. This review should confirm that these licenses allow for sufficient operational flexibility and do not inadvertently infringe upon the IP rights of others, which is crucial in AI where underlying algorithms or datasets might be sourced externally, or where the scope of the license grant does not reasonably contemplate the use of the underlying material in modern technology.
- Outbound Licensing and Risk Management: Carefully assess how the company licenses its technology (including the output of its AI models) to others. It’s important to ensure these agreements safeguard the company’s proprietary technologies and algorithms, providing protection without exposing the company to legal vulnerabilities. AI companies should consider including specific indemnification clauses that address potential legal claims associated with the outputs of their AI models. Moreover, clearly articulated warranties should outline what the technology does and does not guarantee. This approach helps manage customer expectations and mitigate legal risks, ensuring that liability is aligned with the capabilities and intended use of the AI outputs.
- Open Source Software Compliance
- Use and Integration: Investigate the integration and use of open source software (and open source datasets) within the company’s products and whether (and to the extent) open source components have been used in the training of any AI models. AI companies often leverage open-source tools for building algorithms or learning models; hence, it is critical to comply with the respective open-source licenses to prevent any conflicts, particularly with copyleft licenses that could pose challenges to proprietary systems.
- AI-Specific Model Concerns
- Data Training and Model Development: As AI systems are trained using large datasets, the provenance and legality of the sourced data must be verified. This includes ensuring that data used for training AI models, whether proprietary, open-source, or third-party, does not violate any data privacy laws (including emerging regulatory laws preventing the use of certain data in connection with AI systems), copyright restrictions, or statutes directed towards access to and use of computer systems. The risk of embedding biases in AI models also necessitates the review of data acquisition methods and the diversity of data sources.
- Algorithmic Transparency and Patentability: The proprietary nature of algorithms and their eligibility for patent protection require clear documentation and verification. Since AI applications can be a gray area in patent law (a position which varies in different jurisdictions), particularly around the requirement that inventors must be human, detailing the development process and human intellectual contribution to the AI solutions is critical.
Regulatory Landscape for AI
Understanding the regulatory environment is crucial, as it directly impacts how AI companies can operate and scale. This includes navigating:
- Evolving AI Regulation: The EU AI Act is poised to become the world’s first comprehensive AI legislation and is expected to be officially enacted within the next month or two. In the U.S., President Biden advanced federal AI regulation with an Executive Order issued in October 2023, yet states continue to propose their own regulatory measures. Some states and local governments have issued limited AI laws, such as New York City and the State of Illinois. In Asia, the PRC issued interim measures on managing generating AI services that went into effect in August 2023. As new regulatory frameworks continue to involve, it is critical to understand their potential impact.
- Data Protection Laws: In the absence of comprehensive AI legislation, existing laws are serving as the basis for the legal framework surrounding AI. These include GDPR in Europe (soon to be augmented by the EU AI Act), CCPA/CPRA in California, the U.S. Computer Fraud and Abuse Act and Digital Millennium Copyright Act.
- Sector-Specific Regulatory Landscape: AI applications span various sectors, each with its own regulatory demands. For instance, AI in healthcare must comply with HIPAA in the U.S., while autonomous vehicles must navigate an evolving landscape of transportation regulations.
- Company Preparedness: AI companies must demonstrate an understanding of and compliance with sector-specific regulations. This includes regular compliance audits and possibly third-party reviews to assure investors of the company’s commitment to regulatory adherence.
Review of Legal Precedents
Several legal cases have already influenced the IP landscape for AI, setting forth the foundational principles on which future cases will rely to further define legal boundaries. A brief snapshot of the relevant U.S. cases on these issues include:
- Burrow-Giles Lithographic Co. v. Sarony: This case found that a photograph can be a creative work, rather than a mere mechanical reproduction, providing legal guideposts for determining when technology is merely a tool for human expression.
- Alice Corp. v. CLS Bank International: This case addressed the patentability of software and algorithms, setting a precedent that has made obtaining patents on AI-related innovations more challenging.
- hiQ Labs, Inc. v. LinkedIn Corp.: This case explored the legality of scraping publicly accessible data from websites, with the court allowing the practice under certain conditions.
- Google Books Case: This case established precedents on transformative use and fair use, which are particularly relevant when AI systems analyze or repurpose copyrighted material.
- Naruto v. Slater: This case examined whether a monkey that took photographs of himself on a camera was the author and owner of the photographs, with the court ruling that the author of a U.S. copyrighted work must be human.
Practical Strategies for AI Companies
To optimize their IP strategy and attract outside investment, AI companies should:
- Develop Comprehensive IP Management Plans: Implementing systematic approaches to IP creation and protection is crucial. This includes regular IP audits and maintaining detailed records of all IP-related activities, including ownership and indemnification.
- Proactive Licensing Management: Maintaining meticulous records of all licensing agreements and ensuring ongoing compliance is essential, especially with regard to the use of open source software.
- AI Policy and Usage: Establish a company AI policy addressing acceptable AI tools and use parameters. Before using AI-generated content, ensure it is sourced from compliant providers. Diligently review terms of service and privacy policies of AI platforms to avoid using tools with unclear data licensing.
- AI Copyright Infringement Management: Understand the nuances of copyright law, particularly regarding the use of copyrighted works for training AI systems, and assess fair use possibilities to reduce infringement risks.
- Robust Data Privacy and Security Practices: Implement comprehensive data privacy measures such as Privacy by Design, data anonymization, robust data governance, and regular audits.
- Transparent Human Resource Practices Using AI: Ensure transparency in AI’s role in HR processes and maintain oversight to prevent biases and discrimination.
- Meticulous Contracting and Vendor Evaluations: Carefully assess vendors for their compliance with legal standards, particularly concerning AI technologies’ scalability, integration, and support.
- Contractual Provisions: Include specific terms in contracts related to AI usage that mandate disclosure of AI use, ensure compliance with data set licensing, and provide indemnification for IP infringements.
Conclusion
For both AI companies and investors, a thorough understanding of intellectual property rights and the potential legal challenges is essential. Companies must be proactive in managing their IP assets to secure their innovations and protect themselves against potential infringements. This involves rigorous compliance checks, strategic licensing agreements, and meticulous documentation of AI development processes. For investors, conducting detailed due diligence on IP matters is not just about assessing current value but also about understanding the long-term sustainability and legal viability of their investment in AI technology. By collaborating, both parties can ensure that the innovative potential of AI is maximized while navigating the complexities of the legal landscape effectively.
Contributors
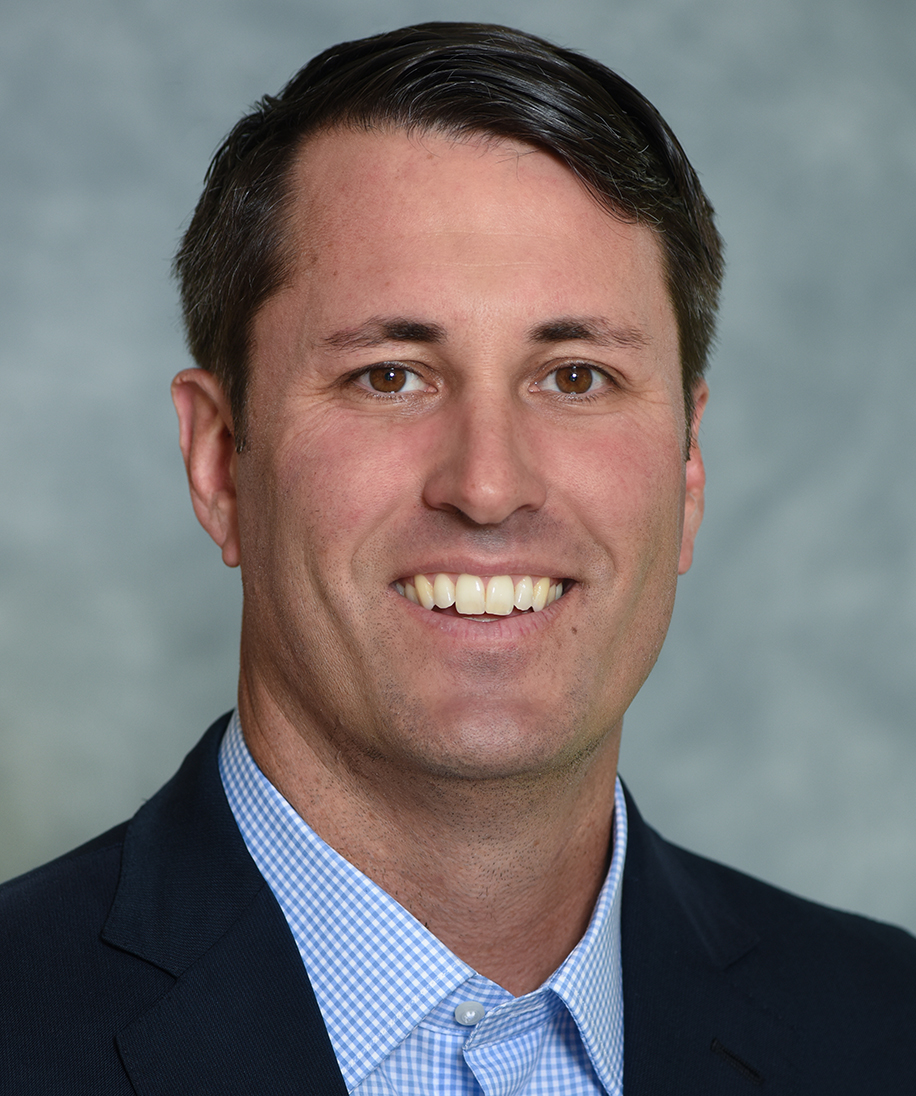
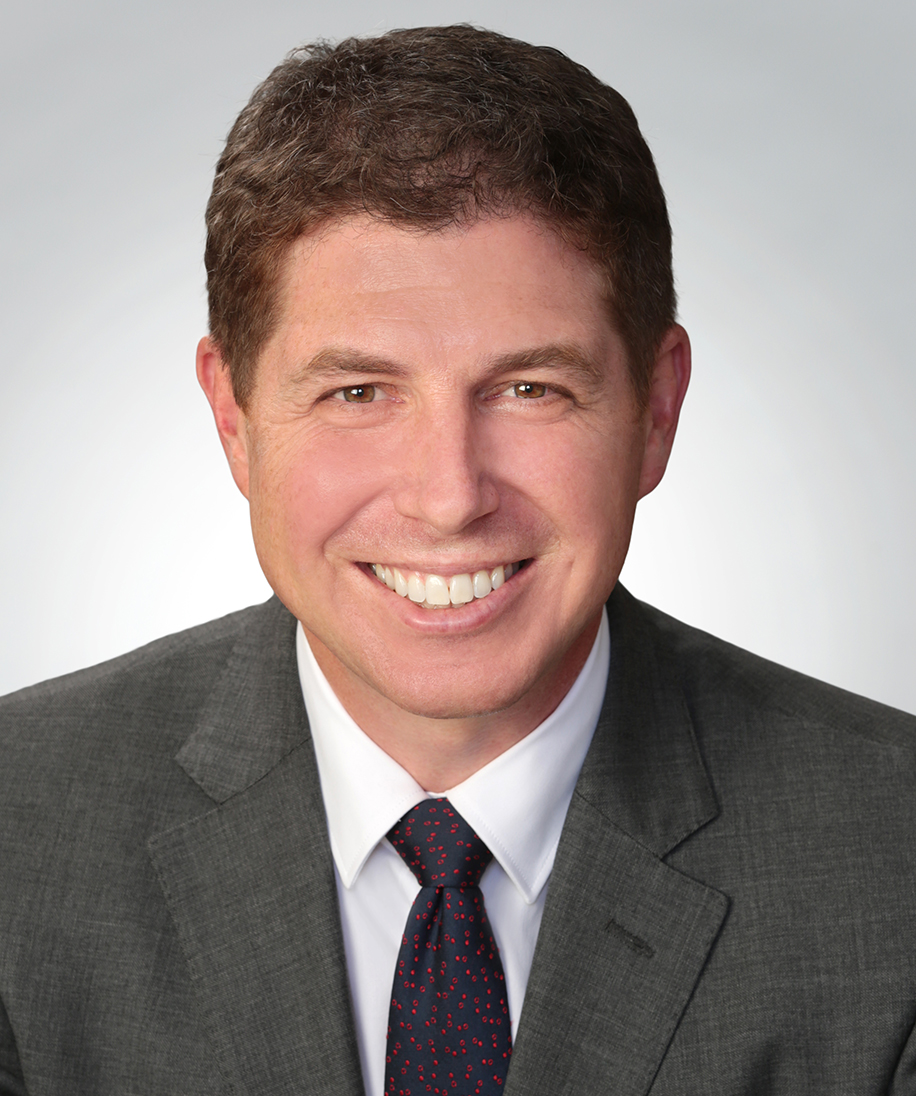
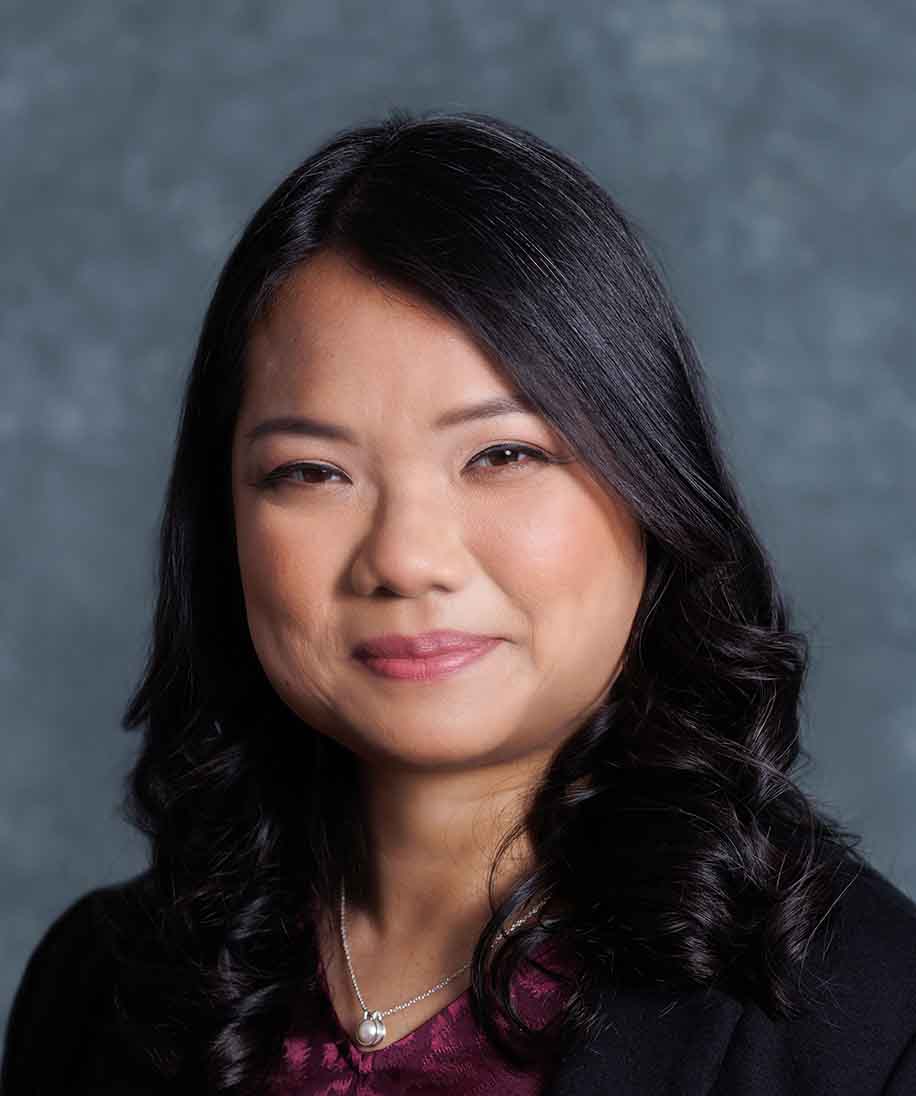
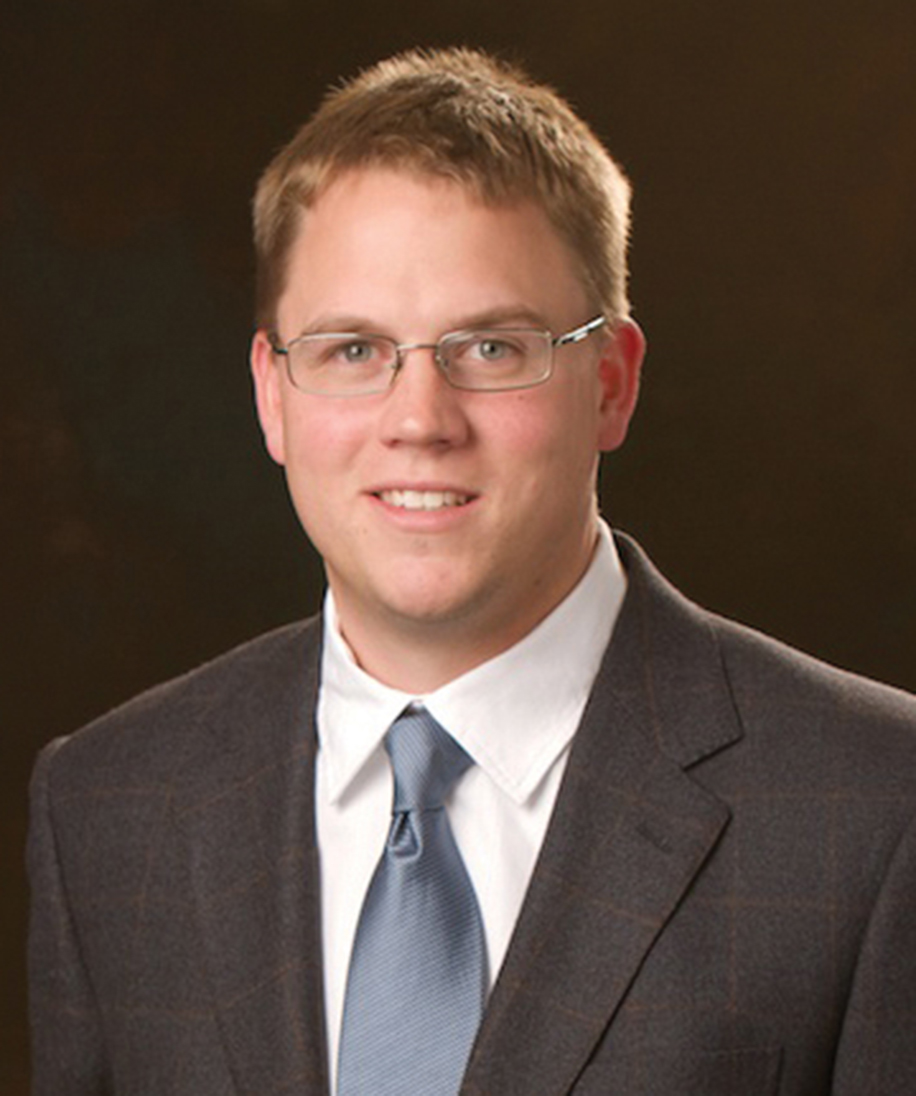
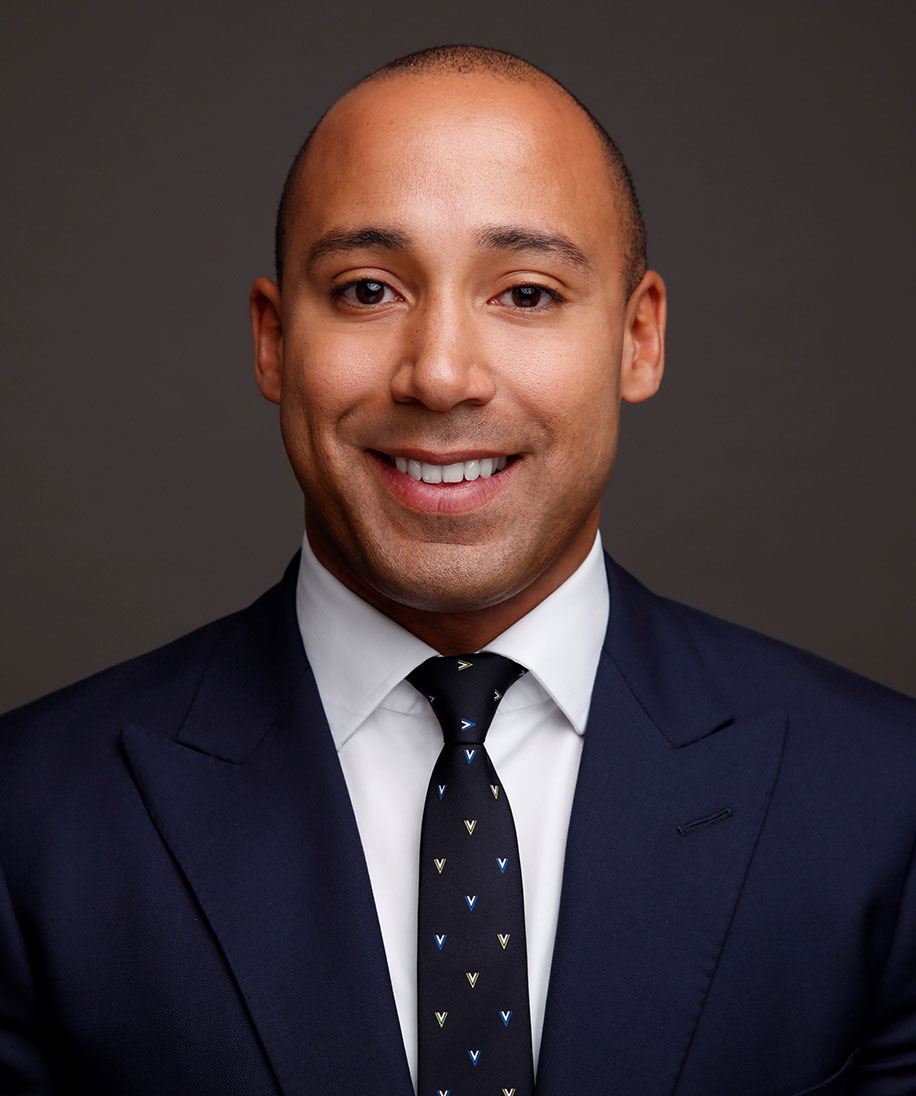
Practice Areas
Data Privacy and Cybersecurity
Privacy and Cybersecurity Solutions Group
Securities and Capital Markets
For More Information
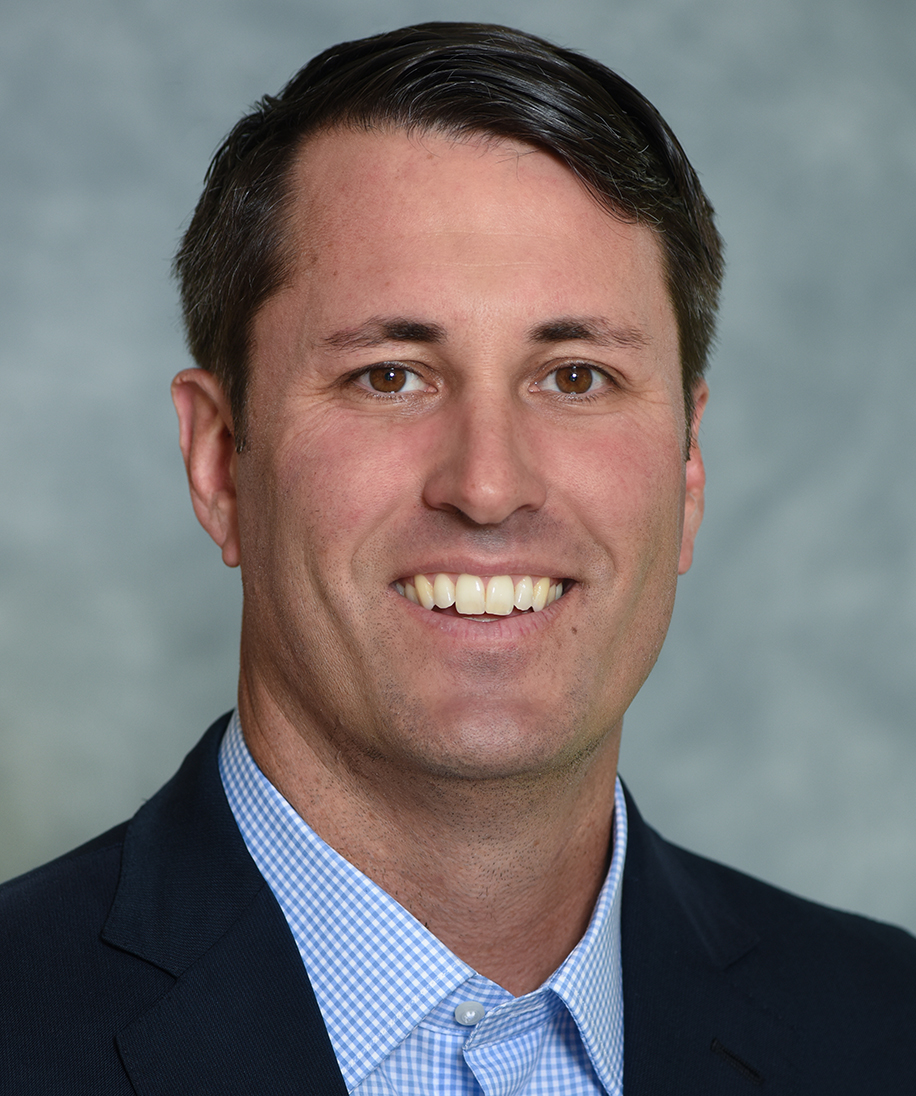
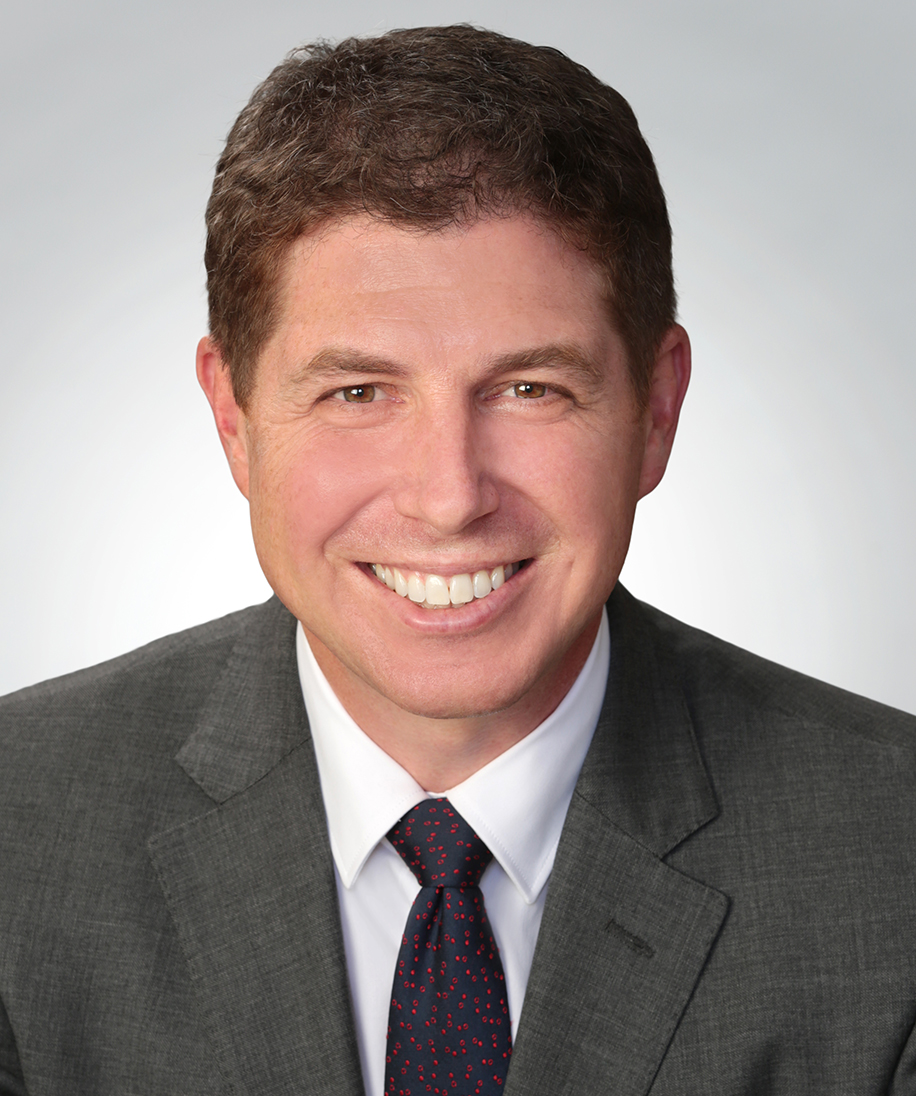
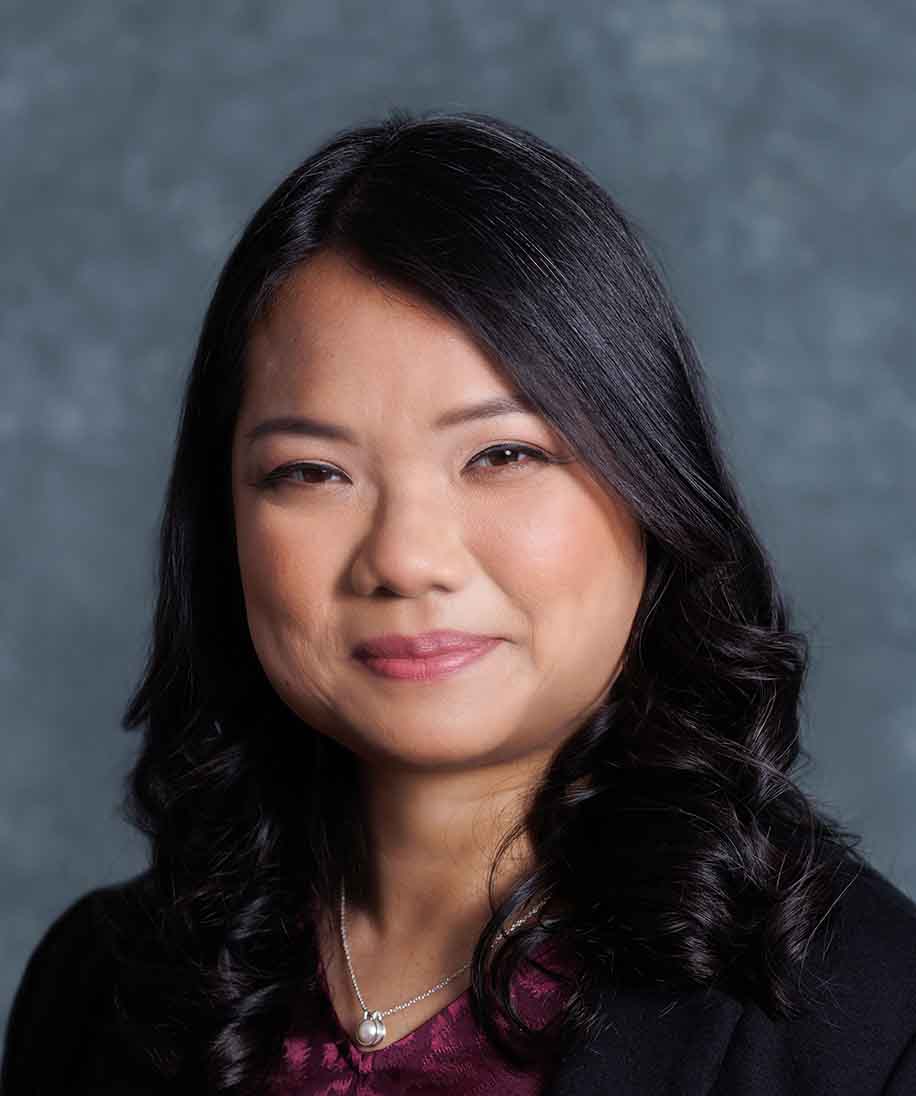
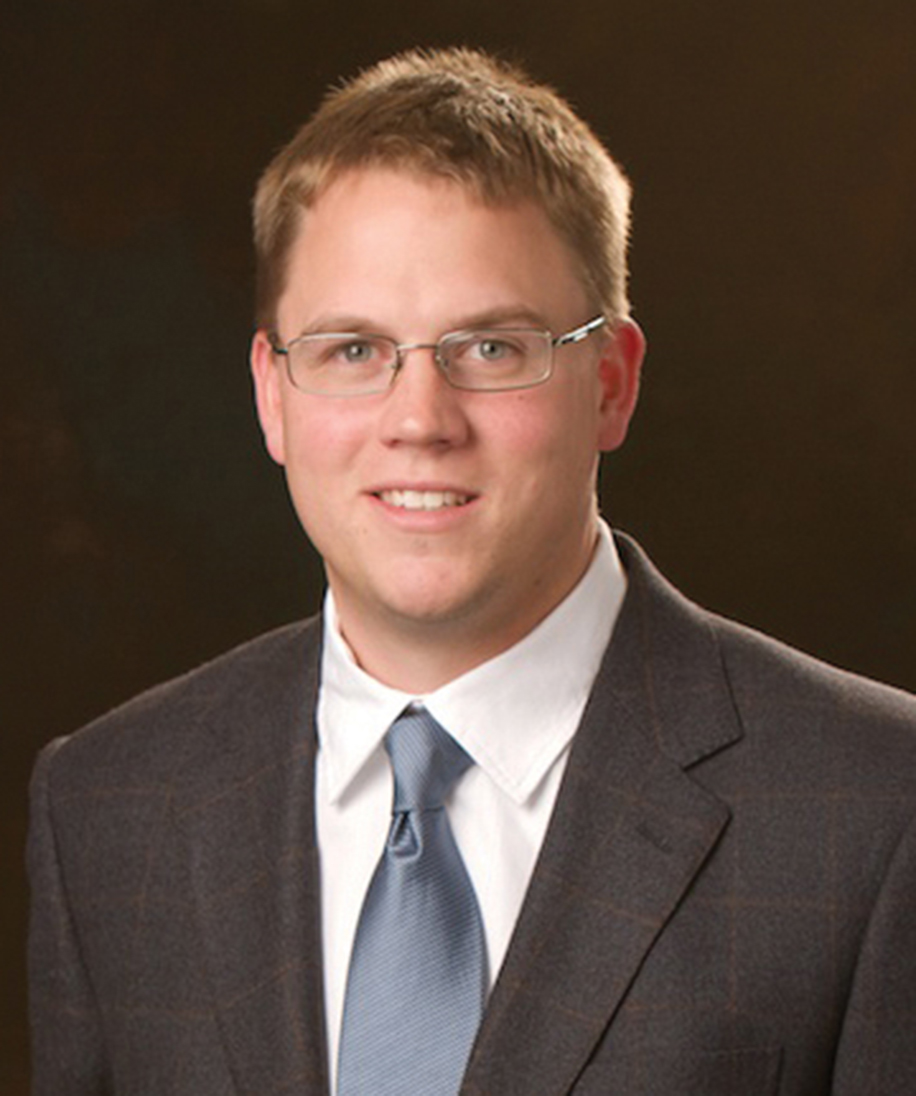
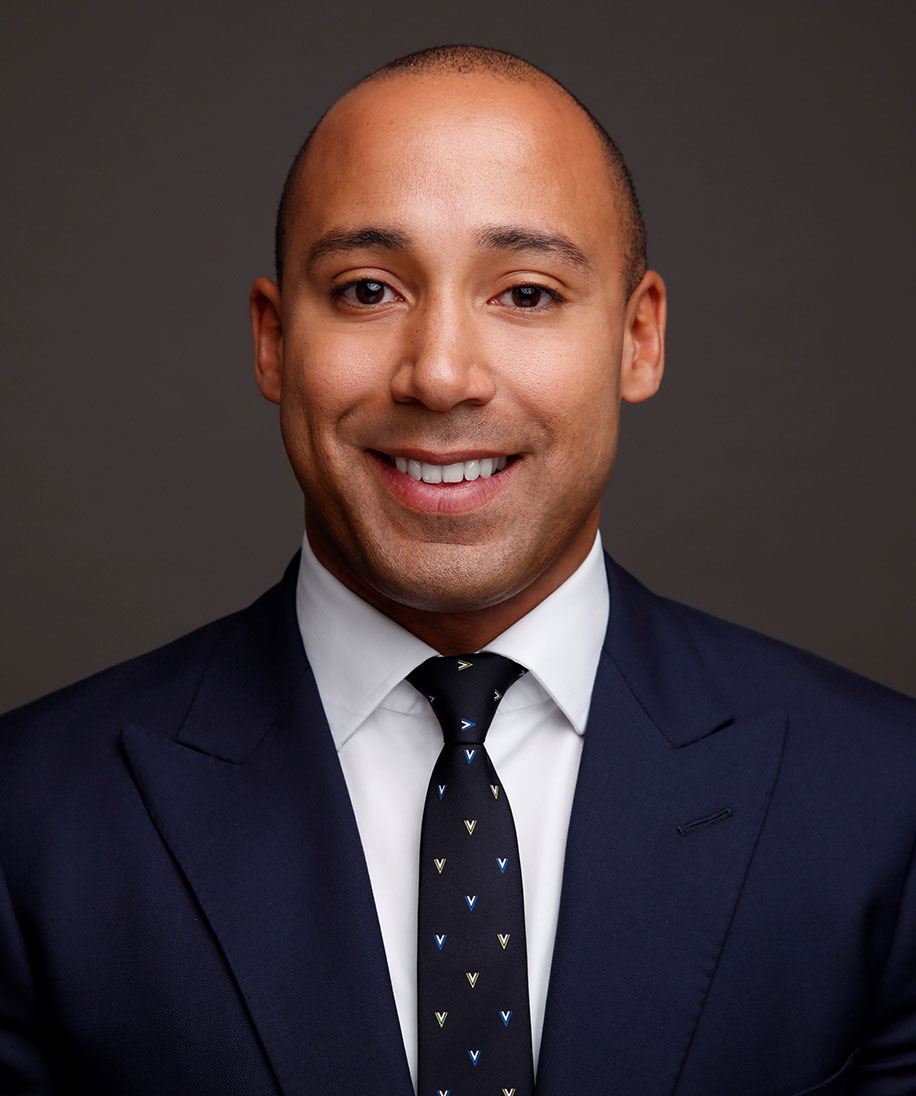